Concepts in Deep Learning
Because deep learning (DL) is a type of machine learning (ML), much of what was described in Concepts in Machine Learning serves as a prologue to the more specific overview of concepts in deep learning provided here. In what follows, we will elaborate on bit more on specific aspects of deep learning algorithms and pipelines.
Neural networks
Neural networks are flexible computational models that can process inputs and produce outputs, with parameters that can be fit or estimated based on data. They represent a type of computational graph inspired by the action of neurons in the brain. The structure of these models reflects a directed network of artificial "neurons" or nodes, in which data are presented as inputs to a network, those inputs are fed through the neural network architecture — which includes one or more hidden layers — and which ultimately produces outputs. This general architecture is represented schematically in the left panel of the figure below. It is conventional to represent neural networks as flowing from left to right, with the input nodes on the far left and the output nodes on the far right. Within the illustration of an entire neural network, we highlight a subset of nodes and edges in red.
Just as the network as a whole includes a set of inputs and outputs, so does each individual node in the network, as illustrated in the right panel of the figure below. Each edge that connects two nodes carries with it an associated weight, indicating the strength of influence between the two nodes. In the network depicted below, for each node
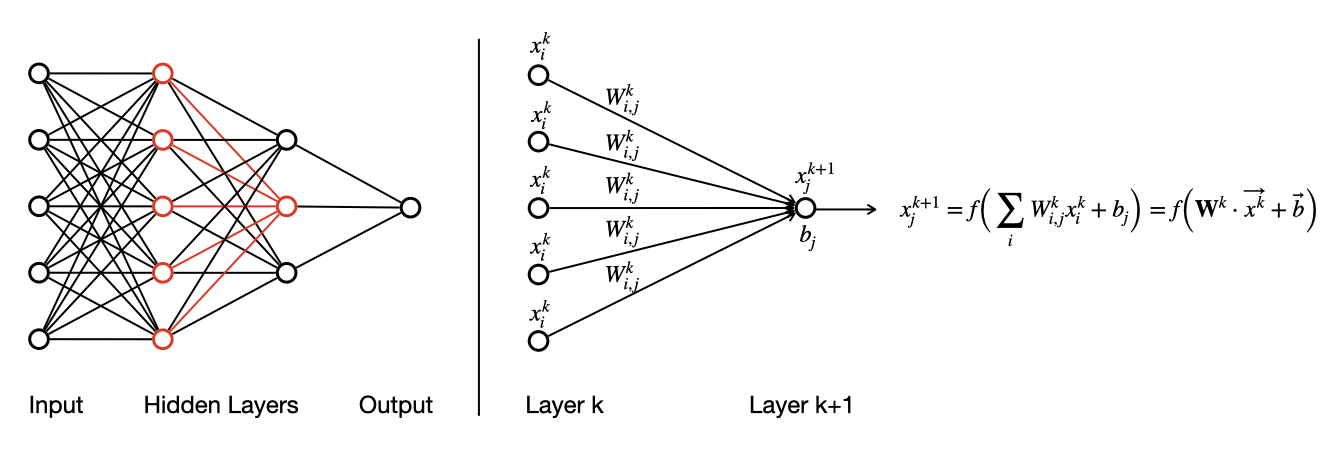
The action of the network is parameterized by the edge weights
A model is trained — that is, its parameters are estimated from data — by making iterative modifications to the parameter values in order to reduce the loss associated with the current set of parameter values. This is typical of many optimization algorithms in scientific computing, where one wishes to move "downhill" along the loss surface. With neural networks, deciding how specifically to change the values of parameters in order to move most quickly downhill is aided by a process known as backpropagation. Leveraging the magic of calculus and a computational technique known as
Designing an appropriate network architecture for a given problem is itself an art and a science, the details of which are beyond the scope of this tutorial. For certain classes of problems, however, there are architectural elements that are regularly used, such as convolutional layers for image processing applications and transformer architectures for large language models. We discuss this in a bit more detail on the following page.